Zhen-Hao Guo and Hai-Cheng Yi, master students of the multilingual information technology lab of the Xinjiang Technical Institute of Physics and Chemistry, Chinese Academy of Sciences, conducted a study on large-scale heterogeneous biologicalmolecular association networkunder the guidance of Prof. Zhu-Hong You. The work which called Construction and Comprehensive Analysis of a Molecular Association Network via lncRNA-miRNA-Disease-Drug-Protein Graph" has been published in Cellsjournal.
This work is the first time to propose the Molecular Association Network (MAN)in the international academic community. The MAN is a network thatsystematically integrated 8 different types of biomolecules (including lncRNA, miRNA, circRNA, mRNA, microbe, disease, Drug and protein) and 18 kinds of associations (including miRNA-disease, circRNA-disease, circRNA-miRNA, disease-mRNA, disease-microbe, drug-disease, drug-mRNA, drug-microbe, drug-protein, lncRNA-disease , lncRNA-mRNA, lncRNA-miRNA, lncRNA-protein, miRNA-drug, miRNA-mRNA, miRNA-protein, mRNA-protein, protein-protein). Based on MAN, a computational framework was established to predict and discover potential associations between biomolecules. How different biomolecules interact with one another to bring about the appropriate cellular activities has been a topic of intense study in the systems biology and genomics community for many years. On the one hand, this work provides new insights for integrating large-scale biological data to construct fast and efficient computational models. On the other hand, it is significant to promote understanding of gene regulation, reveal the molecular mechanism of disease, accelerate the discovery of drug targets, and drug repositioning.
One key issue in the post-genomic era is how to systematically describe the associations between small molecule transcripts or translations inside cells. With the rapid development of high-throughput “omics” technologies, the achieved ability to detect and characterize molecules with other molecule targets opens the possibility of investigating the relationships from a global perspective. However, it is unrealistic to conduct manual experiments based on the large amount of biology data in terms of the high false positives and time, so it is urgent to develop computational prediction tools for wet experiments. In response to this critical problem, the work relies on a variety of isolated biomolecular relationships that have been constructed to integrate a long non-coding RNA, microRNA, circular RNA, messenger RNA, microorganisms, diseases, drugs and proteins. Heterogeneous biomolecular association network. From the perspective of system theory and the holism, a computational framework is proposed to predict potential associations between biomolecules based on node attributes and node behavior. More specifically, each node in MAN can be represented as a low-dimensional and dense vector by combining two kinds of information including the attribute of the node itself (e.g. sequences of ncRNAs and proteins, semantics of diseases and molecular fingerprints of drugs) and the behavior of the node in the complex network (associations with other nodes). Random Forest classifier is trained to classify and predict new interactions or associations between biomolecules. The results show that this method can provide accurate, stable and high coverage prediction results of intermolecular relationships. The proposed method can provide new insight for exploration of the molecular mechanisms of disease and valuable clues for disease treatment." Overall, I think the authors have done a decent job in demonstrating how to integrate as much data as possible into a learning framework to predict novel meaningful associations. The results presented are typical of any applied machine learning paper. "The reviewers of the Cellscommented on this breakthrough.
This work was supported by the National Outstanding Youth Science Fund Project of National Natural Science Foundation of China.
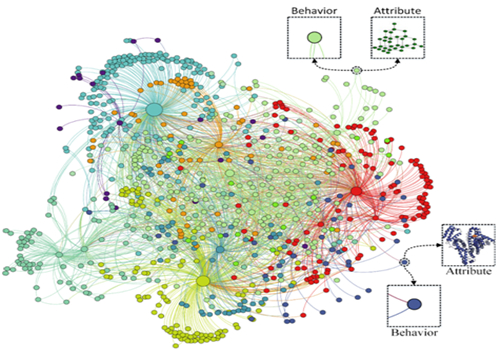
Figure 3. The schematic diagram of Molecular Association Network (MAN).
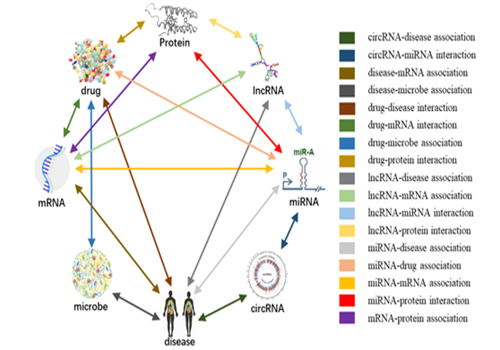
Figure 4. The distribution of molecular associations in MAN.